Blog Articles 31–35
2018
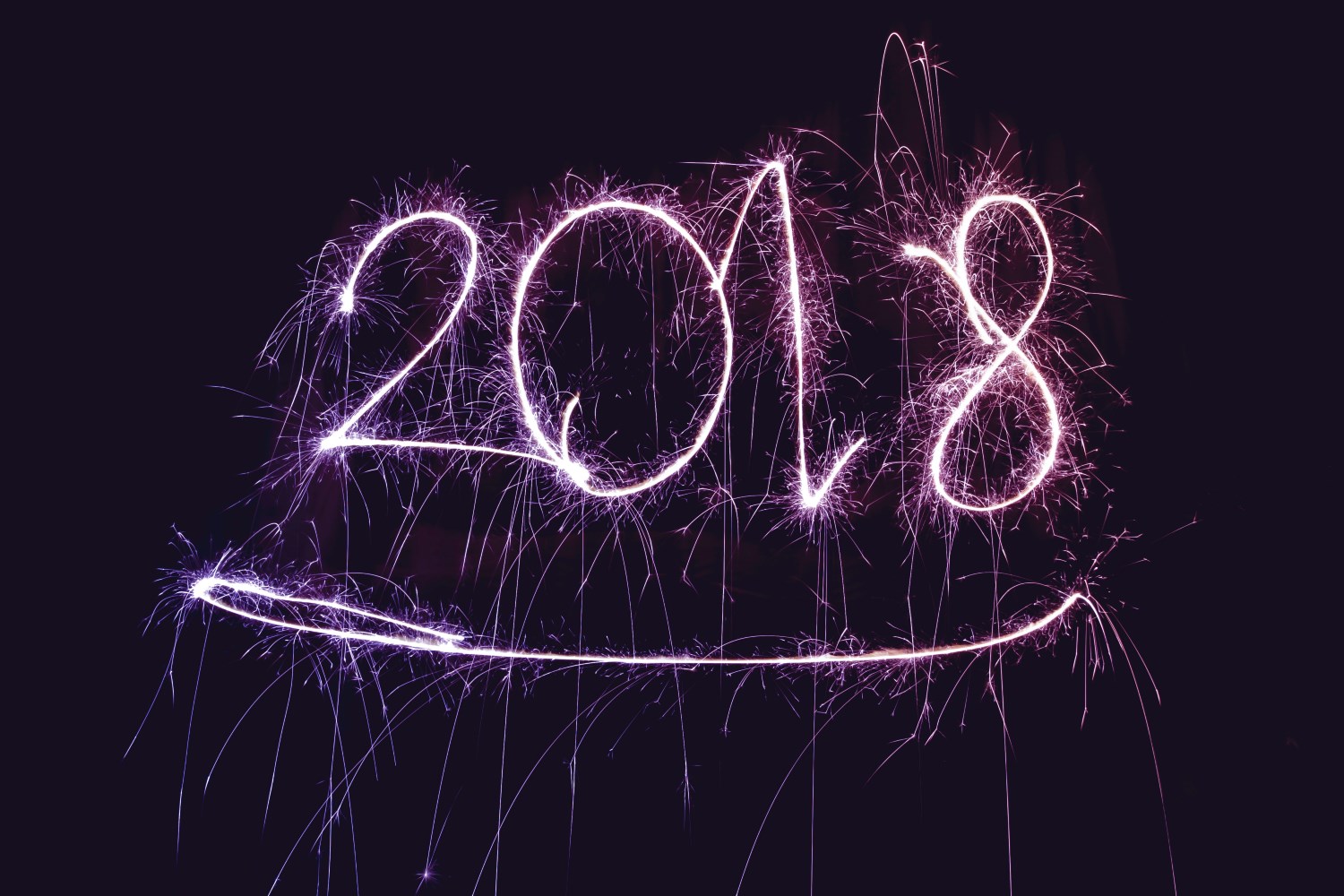
As I’ve done the last two years, it’s time for the annual what-I-did-this-year post! Well, about time; there are a couple more weeks in the year, but I expect their results to be mostly tidying up loose ends of things in this list.
Presented two papers at the inaugural Conference on Fairness, Accountability, and Transparency; one with the PIReTs, and another with Hoda Mehrpouyan and Rezvan Joshaghani.
Published a CHI workshop paper on fairness in privacy tradeoffs with Bart Knijnenburg, Hoda Mehrpouyan, and Rezvan Joshaghani.
Submitted a paper to SIGIR (rejected).
Submitted a proposal to NSF CyberLearning (declined).
Won an NSF CAREER award.
Saw Hamilton (the traveling company in Portland).
Book chapter with Daniel Kluver and Joe Konstan went to press.
Bought a road bike and began recreational distance riding. I got up to being able to do 30mi rides before winding down for the weather.
Co-organized the FairUMAP workshop on fairness in user modeling and personalization with Bamshad Mobasher, Robin Burke, and Bettina Berendt.
Oversaw build-out of the LITERATE prototype and carried out user study with fantastic collaborators Sole Pera and Katherine Wright.
Ran a very successful RecSys 2018 with Sole Pera and our amazing organizing committee.
Published and presented our work on author gender in RecSys 2018.
Taught CS 410/510 (Databases) in both fall and spring.
Taught CS-HU 310, our one-credit database introduction, in the summer.
Substantially improved my response time in grading student work.
Published two workshop papers and contributed to a NRMERA conference talk about the LITERATE project.
Supervised my M.S. student Mucun Tian to his first first-author paper, a work-in-progress piece for the REVEAL workshop on offline evaluation.
Co-organized the second FATERC Workshop on Responsible Recommendation, with more than 50 registered and a full room all day.
With Fernando Diaz and Asia Biega, proposed and had accepted a fairness track for TREC 2019.
With Michael Veale, organized publicity & outreach for ACM FAT* 2019 as Publicity & PR Co-chair.
Began supervising my first Ph.D student, Amifa Raj.
Submitted a proposal to the NSF 2026 IDEA Machine with Sole Pera, Hoda Mehrpouyan, Cathie Olschanowsky, and Elena Sherman.
Sat on commmittees for two successful Ph.D proposals (Ion Madrazo Azpiazu and Kimberley Gardner).
Reviewed a number of papers, though not as many as last year.
Redid my academic visual identity with a website refresh and change of standard font.
I did not submit nearly as many grant proposals this year as last, because I received the CAREER early in the year and needed to focus on getting that research going along with RecSys organization.
Surface Go First Impressions
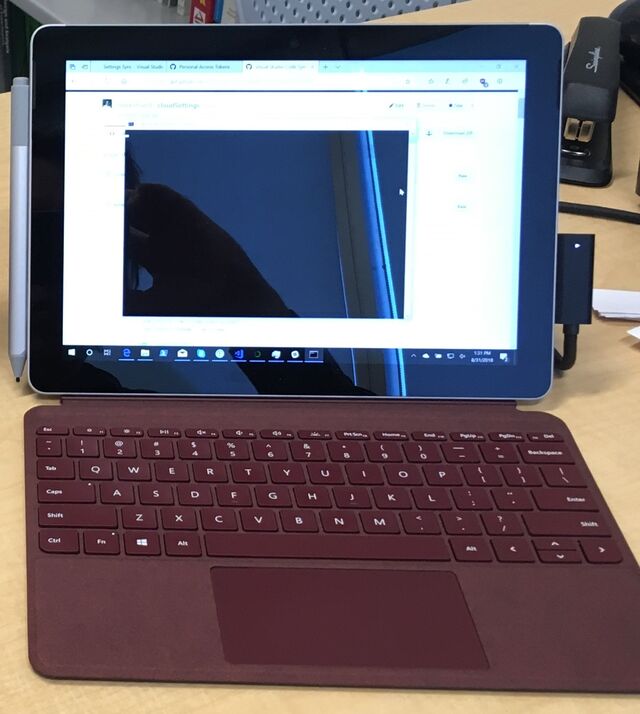
Microsoft has repeatedly been trying to make strides into an entry-level market for its Surface devices, and so far none of them have stuck. There was the Surface RT, which used an incompatible processor and couldn’t run normal Windows software. The Surface 3 used an Atom CPU and didn’t last long. And now they have the Surface Go, a 10” Surface sporting a Pentium processor and full Windows 10.
I have been using the Surface Pro for a few years now. I love them, but have also had some reliability issues: my work SP4 has been glitchy as long as I have had it (display freezes), and my personal device ceased to boot about a year and a half after I bought it. They are on the large side for a lot of tablet use cases — it’s hard to use it as a reading device — but it is fantastic for marking up PDFs and drawing, and I have made significant use of its drawing capabilities in class. The Windows Ink Workspace is very helpful, because I can take a screenshot and start drawing on it to mark up different parts of the query we just ran against the database.
Spending Startup
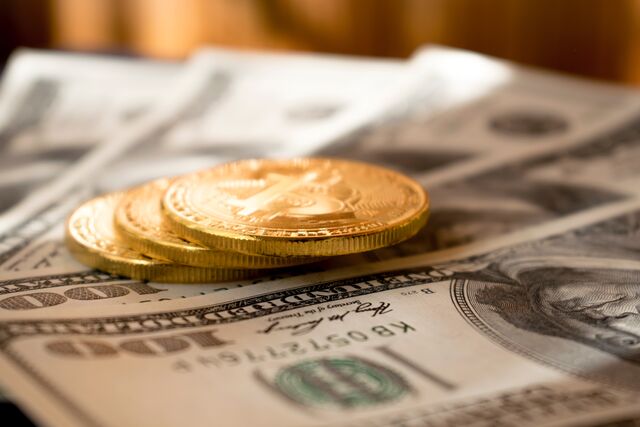
If you are starting a tenure-track research-oriented position at a US university, you should have a startup package to help you get started. When I began as a faculty member, I did not have a clear idea of how to use it effectively; 4 years in, here are some thoughts about good use of startup funds based on my experience and reflection, as well as things I’ve read and heard from others along the way.
This is written from the perspective of a computer science tenure-track position at a mid-tier research-oriented US university. Startup levels, existence, and structure vary between universities and disciplines, so keep that in mind.
Author Gender in Book Recommendations
I’m very pleased that we will be able to present a piece of research we have been working on for some time now at RecSys this year.
In my work on fair recommendation, one of the key questions I want to unravel is how recommender systems interact with issues of representation among content creators. As we work, as a society, to improve representation of historically underrepresented groups — women, racial minories, indigenous peoples, gender minorities, etc. — will recommender systems hinder those efforts? Will ‘get recommended to potential audiences’ be yet another roadblock in the path of authors from disadvantaged groups, or might the recommender aid in the process of exposing new creators to the audiences that will appreciate their work and make them thrive?
In this paper, we (myself, my students Mucun Tian and Imran Kazi, and my colleagues Hoda Mehrpouyan and Daniel Kluver) present our first results on this problem. This work, along with our work on recommender evaluation errors, formed the key preliminary results for my NSF CAREER proposal.
This paper has a few firsts for me. It’s my first fully-Bayesian paper, and is also the first time I have been able to provide complete code to reproduce the experiments and analysis with the manuscript submission.