NSF CAREER award on recommenders, humans, and data
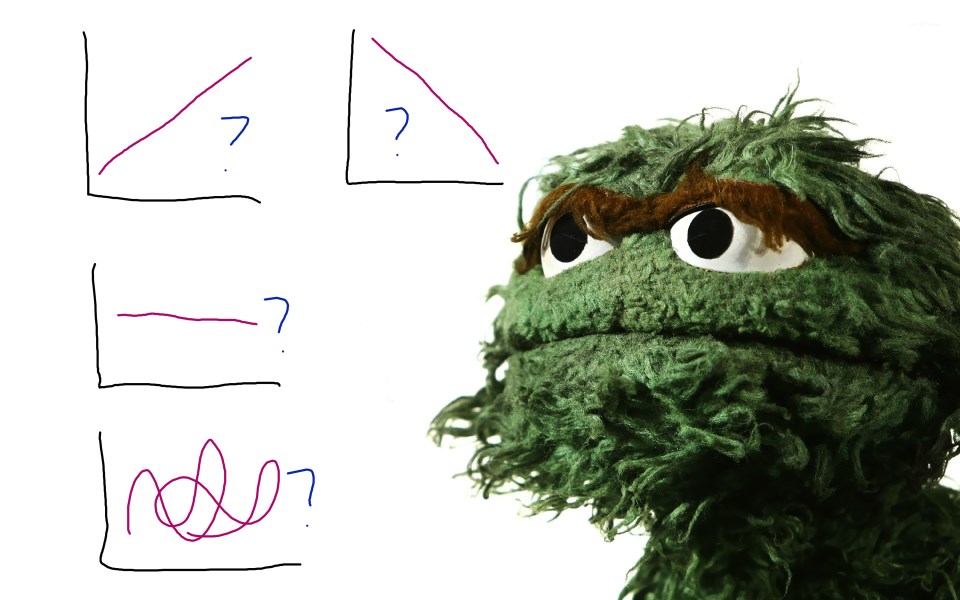
In 2018, I was awarded the NSF CAREER award to study how recommender systems and our evaluations of them respond to the human messiness of their input data. Us computer scientists have long known the principle of ‘garbage in, garbage out’: with bad data, a system will produce bad outputs. But in practice, computing systems can differ a great deal in precisely how they translate such inputs to outputs.
Our goal in this project is to understand that response — to characterize the ‘garbage response curve’ of common recommendation algorithms and surrounding statistical and experimental techniques. For a given type and quantity of garbage (metric/intent mismatch, discriminatory bias, polarized content), we want to understand its impact on recommendations, subsequent human behavior, and the information experiments provide to operators of recommender systems. Systems that recommend products, places, and services are an increasingly common part of everyday life and commerce, making it important to understand how recommendation algorithms affect outcomes for both individual users and larger social groups. To do this, the project team will develop novel methods of simulating users’ behavior based on large-scale historical datasets. These methods will be used to better understand vulnerabilities that underlying biases in training datasets pose to commonly-used machine learning-based methods for building and testing recommender systems, as well as characterize the effectiveness of common evaluation metrics such as recommendation accuracy and diversity given different models of how people interact with recommender systems in practice. The team will publicly release its datasets, software, and novel metrics for the benefit of other researchers and developers of recommender systems. The work also will inform the development of course materials about the social impact of data analytics and computing as well as outreach activities for librarians, who are often in the position of helping information seekers understand the way search engines and other recommender systems affect their ability to get what they need. The work is organized around two main themes. The first will quantify and mitigate the popularity bias and misclassified decoy problems in offline recommender evaluation that tend to lead to popular, known recommendations. To do this, the team will develop simulation-based evaluation models that encode a variety of assumptions about how users select relevant items to buy and rate and use them to quantify the statistical biases these assumptions induce in recommendation quality metrics. They will calibrate these simulations by comparing with existing data sets covering books, research papers, music, and movies. These models and datasets will help drive the second main project around measuring the impact of feature distributions in training data on recommender algorithm accuracy and diversity, while developing bias-resistant algorithms. The team will use data resampling techniques along with the simulation models, extended to model system behavior over time, to evaluate how different algorithms mitigate, propagate, or exacerbate underlying distributional biases through their recommendations, and how those biased recommendations in turn affect future user behavior and experience. Per NSF policy, all published papers are deposited in the NSF Public Access Repository, searchable by award ID; see the list associated with this grant. 2025. Fairness in Information Access: Conceptual Foundations and New Directions. Tutorial presented at Proceedings of the 47th European Conference on Information Retrieval, Apr 6, 2025. DOI 10.1007/978-3-031-88720-8_41. 2024. It’s Not You, It’s Me: The Impact of Choice Models and Ranking Strategies on Gender Imbalance in Music Recommendation. Short paper in Proceedings of the 18th ACM Conference on Recommender Systems (RecSys ’24), Oct 14, 2024. ACM, pp. 884–889. DOI 10.1145/3640457.3688163. arXiv:2409.03781 [cs.IR]. NSF PAR 10568004. Acceptance rate: 22%. Cited 3 times. Cited 2 times. 2024. Not Just Algorithms: Strategically Addressing Consumer Impacts in Information Retrieval. In Proceedings of the 46th European Conference on Information Retrieval (ECIR ’24, IR for Good track), Mar 24–28, 2024. Lecture Notes in Computer Science 14611:314–335. DOI 10.1007/978-3-031-56066-8_25. NSF PAR 10497110. Acceptance rate: 35.9%. Cited 13 times. Cited 5 times. 2024. Towards Optimizing Ranking in Grid-Layout for Provider-side Fairness. In Proceedings of the 46th European Conference on Information Retrieval (ECIR ’24, IR for Good track), Mar 24–28, 2024. Lecture Notes in Computer Science 14612:90–105. DOI 10.1007/978-3-031-56069-9_7. NSF PAR 10497109. Acceptance rate: 35.9%. Cited 3 times. Cited 2 times. 2024. Multiple Testing for IR and Recommendation System Experiments. Short paper in Proceedings of the 46th European Conference on Information Retrieval (ECIR ’24), Mar 24–28, 2024. Lecture Notes in Computer Science 14610:449–457. DOI 10.1007/978-3-031-56063-7_37. NSF PAR 10497108. Acceptance rate: 24.3%. Cited 3 times. 2023. Towards Measuring Fairness in Grid Layout in Recommender Systems. Presented at the 6th FAccTrec Workshop on Responsible Recommendation at RecSys 2023 (peer-reviewed but not archived). arXiv:2309.10271 [cs.IR]. Cited 1 time. 2023. Candidate Set Sampling for Evaluating Top-N Recommendation. In Proceedings of the 22nd IEEE/WIC International Conference on Web Intelligence and Intelligent Agent Technology (WI-IAT ’23), Oct 26–29, 2023. pp. 88-94. DOI 10.1109/WI-IAT59888.2023.00018. arXiv:2309.11723 [cs.IR]. NSF PAR 10487293. Acceptance rate: 28%. Cited 6 times. Cited 1 time. 2024. Distributionally-Informed Recommender System Evaluation. Transactions on Recommender Systems 2(1) (March 2024; online Aug 4, 2023), 6:1–27. DOI 10.1145/3613455. arXiv:2309.05892 [cs.IR]. NSF PAR 10461937. Cited 17 times. Cited 11 times. 2023. Patterns of Gender-Specializing Query Reformulation. Short paper in Proceedings of the 46th International ACM SIGIR Conference on Research and Development in Information Retrieval (SIGIR ’23), Jul 23, 2023. pp. 2241–2245. DOI 10.1145/3539618.3592034. arXiv:2304.13129. NSF PAR 10423689. Acceptance rate: 25.1%. Cited 4 times. Cited 1 time. 2023. Inference at Scale: Significance Testing for Large Search and Recommendation Experiments. Short paper in Proceedings of the 46th International ACM SIGIR Conference on Research and Development in Information Retrieval (SIGIR ’23), Jul 23, 2023. pp. 2087–2091. DOI 10.1145/3539618.3592004. arXiv:2305.02461. NSF PAR 10423691. Acceptance rate: 25.1%. Cited 4 times. Cited 1 time. 2023. Much Ado About Gender: Current Practices and Future Recommendations for Appropriate Gender-Aware Information Access. In Proceedings of the 2023 Conference on Human Information Interaction and Retrieval (CHIIR ’23), Mar 19, 2023. pp. 269–279. DOI 10.1145/3576840.3578316. arXiv:2301.04780. NSF PAR 10423693. Acceptance rate: 39.4%. Cited 25 times. Cited 13 times. 2022. Matching Consumer Fairness Objectives & Strategies for RecSys. Presented at the 5th FAccTrec Workshop on Responsible Recommendation at RecSys 2022 (peer-reviewed but not archived). arXiv:2209.02662 [cs.IR]. Cited 5 times. Cited 3 times. 2022. Fire Dragon and Unicorn Princess: Gender Stereotypes and Children’s Products in Search Engine Responses. In SIGIR eCom ’22, Jul 15, 2022. 9 pp. DOI 10.48550/arXiv.2206.13747. arXiv:2206.13747 [cs.IR]. Cited 13 times. Cited 6 times. 2022. Measuring Fairness in Ranked Results: An Analytical and Empirical Comparison. In Proceedings of the 45th International ACM SIGIR Conference on Research and Development in Information Retrieval (SIGIR ’22), Jul 11, 2022. pp. 726–736. DOI 10.1145/3477495.3532018. NSF PAR 10329880. Acceptance rate: 20%. Cited 79 times. Cited 54 times. 2022. Fairness in Information Access Systems. Foundations and Trends® in Information Retrieval 16(1–2) (July 2022), 1–177. DOI 10.1561/1500000079. arXiv:2105.05779 [cs.IR]. NSF PAR 10347630. Impact factor: 8. Cited 221 times. Cited 103 times. 2022. The Multisided Complexity of Fairness in Recommender Systems. AI Magazine 43(2) (June 2022), 164–176. DOI 10.1002/aaai.12054. NSF PAR 10334796. Cited 39 times. Cited 22 times. 2022. Fairness in Recommender Systems. In Recommender Systems Handbook (3rd edition). Francesco Ricci, Lior Roach, and Bracha Shapira, eds. Springer-Verlagpp. 679–707. DOI 10.1007/978-1-0716-2197-4_18. ISBN 978-1-0716-2196-7. Cited 53 times. Cited 22 times. 2021. Evaluating Recommenders with Distributions. In Proceedings of the RecSys 2021 Workshop on Perspectives on the Evaluation of Recommender Systems (RecSys ’21), Sep 25, 2021. Cited 2 times. 2021. Baby Shark to Barracuda: Analyzing Children’s Music Listening Behavior. In RecSys 2021 Late-Breaking Results (RecSys ’21), Sep 26, 2021. pp. 639–644. DOI 10.1145/3460231.3478856. NSF PAR 10316668. Cited 15 times. Cited 6 times. 2021. Pink for Princesses, Blue for Superheroes: The Need to Examine Gender Stereotypes in Kids’ Products in Search and Recommendations. In Proceedings of the 5th International and Interdisciplinary Workshop on Children & Recommender Systems (KidRec ’21), at IDC 2021, Jun 27, 2021. arXiv:2105.09296. NSF PAR 10335669. Cited 10 times. Cited 6 times. 2021. Estimation of Fair Ranking Metrics with Incomplete Judgments. In Proceedings of The Web Conference 2021 (TheWebConf 2021), Apr 19, 2021. ACM, pp. 1065–1075. DOI 10.1145/3442381.3450080. arXiv:2108.05152. NSF PAR 10237411. Acceptance rate: 21%. Cited 54 times. Cited 38 times. 2021. Exploring Author Gender in Book Rating and Recommendation. User Modeling and User-Adapted Interaction 31(3) (February 2021), 377–420. DOI 10.1007/s11257-020-09284-2. arXiv:1808.07586v2. NSF PAR 10218853. Impact factor: 4.412. Cited 210 times (shared with RecSys18. Cited 115 times (shared with RecSys18. 2020. LensKit for Python: Next-Generation Software for Recommender Systems Experiments. In Proceedings of the 29th ACM International Conference on Information and Knowledge Management (CIKM ’20, Resource track), Oct 21, 2020. ACM, pp. 2999–3006. DOI 10.1145/3340531.3412778. arXiv:1809.03125 [cs.IR]. NSF PAR 10199450. No acceptance rate reported. Cited 132 times. Cited 82 times. 2020. Evaluating Stochastic Rankings with Expected Exposure. In Proceedings of the 29th ACM International Conference on Information and Knowledge Management (CIKM ’20), Oct 21, 2020. ACM, pp. 275–284. DOI 10.1145/3340531.3411962. arXiv:2004.13157 [cs.IR]. NSF PAR 10199451. Acceptance rate: 20%. Nominated for Best Long Paper. Cited 205 times. Cited 179 times. 2020. Comparing Fair Ranking Metrics. Presented at the 3rd FAccTrec Workshop on Responsible Recommendation at RecSys 2020 (peer-reviewed but not archived). arXiv:2009.01311 [cs.IR]. Cited 41 times. Cited 30 times. 2020. Estimating Error and Bias in Offline Evaluation Results. Short paper in Proceedings of the 2020 Conference on Human Information Interaction and Retrieval (CHIIR ’20), Mar 14, 2020. ACM, 5 pp. DOI 10.1145/3343413.3378004. arXiv:2001.09455 [cs.IR]. NSF PAR 10146883. Acceptance rate: 47%. Cited 15 times. Cited 11 times. 2019. StoryTime: Eliciting Preferences from Children for Book Recommendations. Demo recorded in Proceedings of the 13th ACM Conference on Recommender Systems (RecSys ’19), Sep 19, 2019. 2 pp. DOI 10.1145/3298689.3347048. NSF PAR 10133610. Cited 19 times. Cited 10 times. 2018. Monte Carlo Estimates of Evaluation Metric Error and Bias. Computer Science Faculty Publications and Presentations 148, Boise State University. Presented at the REVEAL 2018 Workshop on Offline Evaluation for Recommender Systems at RecSys 2018. DOI 10.18122/cs_facpubs/148/boisestate. NSF PAR 10074452. Cited 1 time. Cited 1 time. We have given a tutorial on fairness in IR & recommendation in multiple settings. 2019. Fairness and Discrimination in Recommendation and Retrieval. Tutorial presented at Proceedings of the 13th ACM Conference on Recommender Systems (RecSys ’19), Sep 19, 2019. pp. 576–577. DOI 10.1145/3298689.3346964. Cited 53 times. Cited 41 times. 2019. Fairness and Discrimination in Retrieval and Recommendation. Tutorial presented at Proceedings of the 42nd International ACM SIGIR Conference on Research and Development in Information Retrieval (SIGIR ’19), Jul 21, 2019. pp. 1403–1404. DOI 10.1145/3331184.3331380. Cited 59 times. Cited 41 times. I am one of the organizers of the TREC Fairness Track; my participation in this is funded by the grant. I co-organized the Workshop on Fairness, Accountability, Confidentiality, Transparency, and Safety in Information Retrieval. 2019. FACTS-IR: Fairness, Accountability, Confidentiality, Transparency, and Safety in Information Retrieval. SIGIR Forum 53(2) (December 2019), 20–43. DOI 10.1145/3458553.3458556. Cited 52 times. Cited 19 times. 2019. Workshop on Fairness, Accountability, Confidentiality, Transparency, and Safety in Information Retrieval (FACTS-IR). Meeting summary in Proceedings of the 42nd International ACM SIGIR Conference on Research and Development in Information Retrieval (SIGIR ’19), Jul 21, 2019. ACM. DOI 10.1145/3331184.3331644. Cited 6 times. These papers were written prior to the project period, and establish preliminary results that helped secure the grant. 2018. Exploring Author Gender in Book Rating and Recommendation. In Proceedings of the 12th ACM Conference on Recommender Systems (RecSys ’18), Oct 3, 2018. ACM, pp. 242–250. DOI 10.1145/3240323.3240373. arXiv:1808.07586v1 [cs.IR]. Acceptance rate: 17.5%. Citations reported under UMUAI21. Citations reported under UMUAI21. 2017. Sturgeon and the Cool Kids: Problems with Random Decoys for Top-N Recommender Evaluation. In Proceedings of the 30th International Florida Artificial Intelligence Research Society Conference (Recommender Systems track), May 29, 2017. AAAI, pp. 639–644. No acceptance rate reported. Cited 25 times. Cited 11 times. I have three planned educational activities as a part of this project: See the Library Training page for details on library training, and on scheduling a training for your library. I have given the following: This material is based upon work supported by the National Science Foundation under Grant No. IIS 17-51278. Any opinions, findings, and conclusions or recommendations expressed in this material are those of the author(s) and do not necessarily reflect the views of the National Science Foundation.Project Abstract
Links
Research Outcomes
Published Papers and Outputs
Recommendation Tutorial
TREC Track
FACTS-IR Workshop
Preliminary Work
Educational Outcomes
Library Training
Credits