Hamlet and IR Social Impact
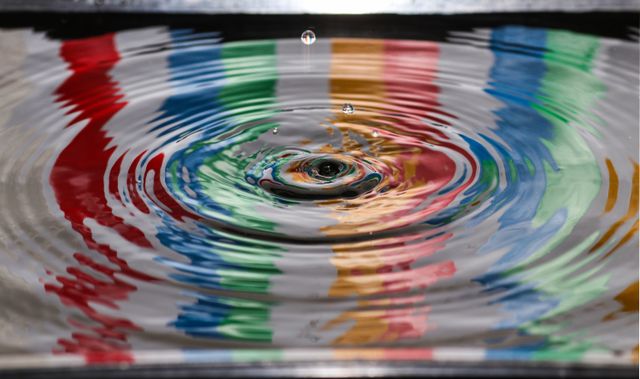
There are more intervention strategies in heaven and earth, Horatio, than are dreamt of in your philosophy.
— Hamlet, sort-of
Tomorrow, I will be presenting our paper in the IR4Good track at ECIR 2024 on strategic IR impact interventions.
From my side, this paper grew out of a couple of things: