Leveling Up Call and Video Quality
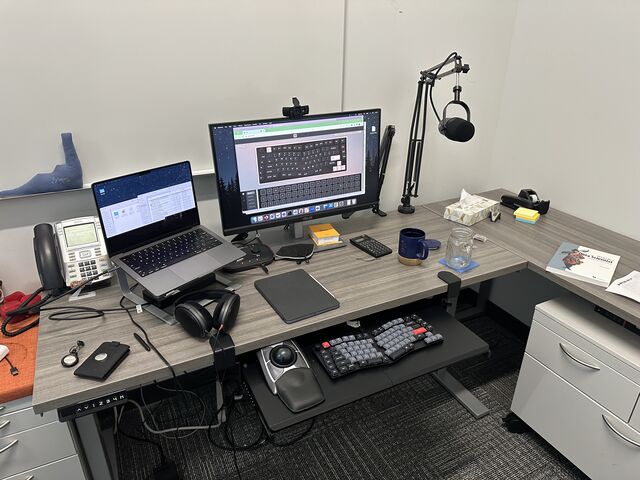
I often get comments, and sometimes questions, about my Zoom and video lecture setup in my office. In this post, I want to talk a bit about it, my choice of equipment, and things that can improve the quality of video recordings, remote teaching, and videoconferences.
There are a few key pieces to this: