Late Work
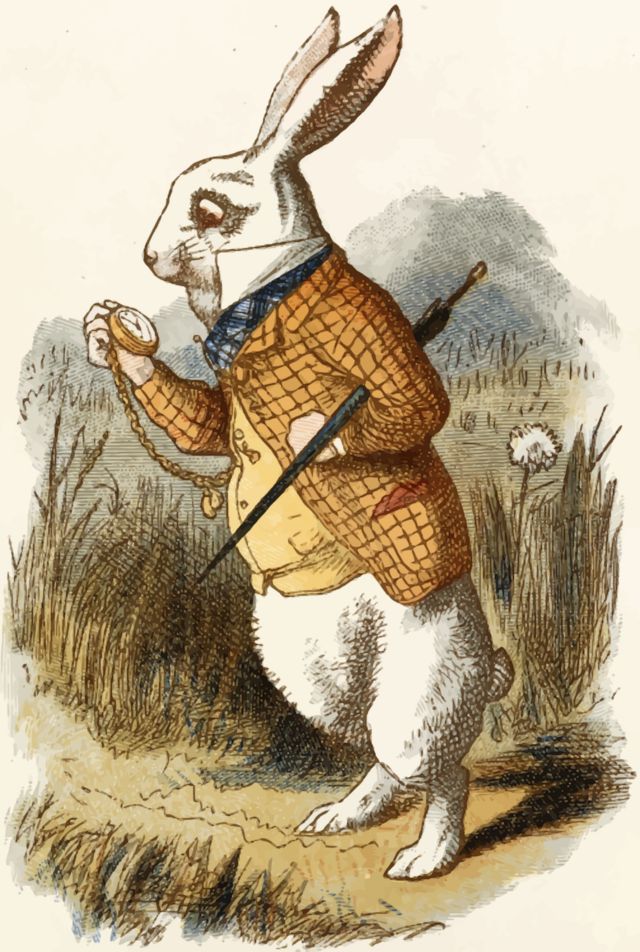
As long as I have been teaching, I’ve used a “late day” policy in most of my classes. I designed this policy after learning about universal course design while taking Preparing Future Faculty at the University of Minnesota, but I don’t think I’ve ever publicly written down the motivations and design of this policy. So here you go!