Presenting at FAT*
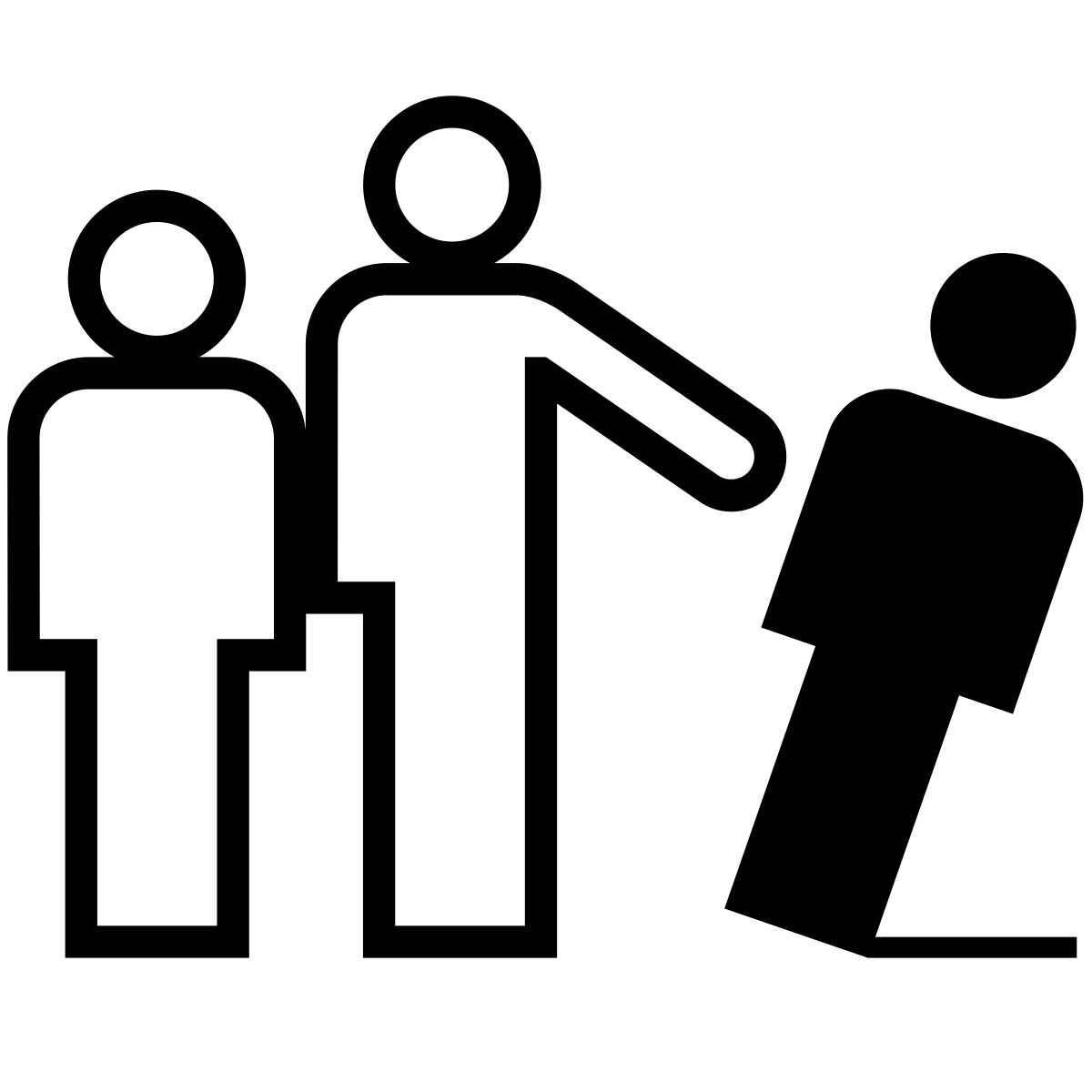
As you’re hopefully well aware if you follow my Twitter, the end of this month will bring the first Conference on Fairness, Accountability, and Transparency (FAT*). It’s been an honor to be involved in some of the planning for this conference series; it is also a great honor to have two papers in the first edition. Algorithmic fairness is the main focus of my research agenda for the next several years.
Fair Privacy
The first of these papers is Privacy for All, a position paper with my colleague Hoda Mehrpouyan and her student Rezvan Joshaghani. This paper arose out of a number of discussions Hoda and I were having about how our research topics and expertise might connect.
In this paper, we discuss the intersection of fairness and privacy, and identify a number of open questions regarding the fairness of privacy protections and the disparate impact of privacy risks. Fairness has been considered in some of the privacy literature — for example, certain fairness properties are part of the design goals for differential privacy — but there has been very little research (that we have been able to find, at any rate) on how these concepts interact in practice.
If already-vulnerable groups obtain less protection from privacy schemes, or pay a higher cost for obtaining that protection, that would be a bad thing. We want to see (and carry out) research to better understand how privacy risks and protections are distributed across society.
Hoda and I will be presenting this paper in the first paper session. We may even present it together! Though likely not in unison.
2018. Privacy for All: Ensuring Fair and Equitable Privacy Protections. In Proceedings of the 1st Conference on Fairness, Accountability and Transparency (FAT* 2018), Feb 23, 2018. PMLR, Proceedings of Machine Learning Research 81:35–47. Acceptance rate: 24%. Cited 107 times. Cited 81 times.
, , and .Disparate Effectiveness of Recommendations
The second is All The Cool Kids, How Do They Fit In? with Sole Pera and our students in PIReT.
In this follow-up to our RecSys 2017 poster, we demonstrate that recommender systems do not provide the same accuracy of recommendations, as we are able to measure, to all demographic groups of their users. We found:
- In the MovieLens 1M and LastFM 1K data sets, men receive better recommendations than women.
- In the LastFM 360K data set, old (50+) and young (under 18) receive better recommendations than other age groups.
- These differences persist after controlling for the number of movies or songs a user has rated or played.
- The MovieLens differences diminish, but do not seem to go away entirely, when we resample data to have the same number of men and women (but we need more nuanced statistics to better understand this difference).
- Correcting for popularity bias can significantly change the demographic distribution of effectiveness, indicating a tradeoff in correcting for different misfeatures of recommender evaluation.
Demonstrating differences like these is the first step in understanding who benefits from recommender systems (and other information systems). Are our systems delivering benefit for all their users? Are we ok with that?
I’ll be presenting this paper in the last session.
2018. All The Cool Kids, How Do They Fit In?: Popularity and Demographic Biases in Recommender Evaluation and Effectiveness. In Proceedings of the 1st Conference on Fairness, Accountability and Transparency (FAT* 2018), Feb 23, 2018. PMLR, Proceedings of Machine Learning Research 81:172–186. Acceptance rate: 24%. Cited 324 times. Cited 226 times.
, , , , , , and .