Readings and Schedule
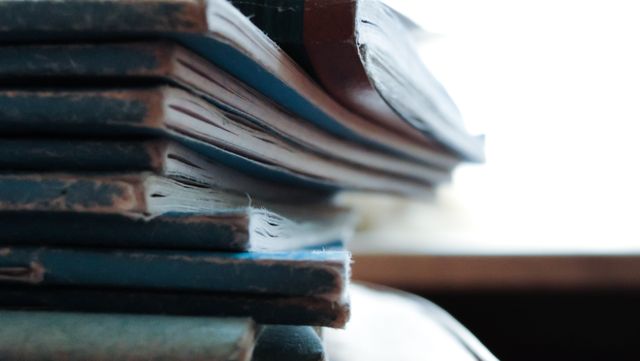
This is a reading-intensive course. Before class (by noon), e-mail me a 3-sentence summary of the paper’s contributions and 1 thing you learned from reading it. Don’t spend long on this e-mail. Probability theory — we will use probability notation a lot. Python for Data Analysis, available in the library’s e-book platform. S. Keshav. 2007. How to Read a Paper. SIGCOMM Comput. Commun. Rev. 37, 3 (July 2007), 83–84. doi:10.1145/1273445.1273458. How to Read a Research Paper by Michael Mitzenmacher. Batya Friedman and Helen Nissenbaum. 1996. Bias in Computer Systems. ACM Transactions on Information Systems 14, 3 (July 1996), 330–347. doi:10.1145/230538.230561. Cynthia Dwork, Moritz Hardt, Toniann Pitassi, Omer Reingold, and Richard Zemel. 2012. Fairness Through Awareness. In Proceedings of the 3rd Innovations in Theoretical Computer Science Conference (ITCS ’12), 214–226. doi:10.1145/2090236.2090255. No class meetings while I am at ACM FAT*. Use the time you would spend in class to watch livestreams of FAT* sessions. Feel free to meet in the classroom to watch some together. I also recommend getting ahead on next week’s reading. This week will have 3 topics divided across its 2 days: Takeaways from FAT* (your livestream viewing and my attendance) Discuss a paper by Barocas and Selbst: Solon Barocas and Andrew D. Selbst. 2016. Big Data’s Disparate Impact. California Law Review 104, 3 (2016), 671. doi:10.2139/ssrn.2477899. This is a long paper by page count, but it is not as nearly so mathematically dense as some of the other papers we are reading. Discuss assignment papers and clarify questions or doubts Shira Mitchell, Eric Potash, Solon Barocas, Alexander D’Amour, and Kristian Lum. 2018. Prediction-Based Decisions and Fairness: A Catalogue of Choices, Assumptions, and Definitions. arXiv [stat.AP]. urn:arxiv:1811.07867. Kristian Lum and William Isaac. 2016. To predict and serve? Significance 13, 5 (October 2016), 14–19. doi:10.1111/j.1740-9713.2016.00960.x. One of the following papers: Danielle Ensign, Sorelle A. Friedler, Scott Neville, Carlos Scheidegger, Suresh Venkatasubramanian. 2018. Runaway Feedback Loops in Predictive Policing. In Proceedings of the Conference on Fairness, Accountability, and Transparency, PMLR 81:160–171. [video] Danielle Ensign, Sorelle A. Friedler, Scott Neville, Carlos Scheidegger, Suresh Venkatasubramanian. 2017. Runaway Feedback Loops in Predictive Policing. In FAT/ML 2017. urn:arXiv:1706.09847v1 [video] Chelsea Barabas, Madars Virza, Karthik Dinakar, Joichi Ito, Jonathan Zittrain. 2018. Interventions over Predictions: Reframing the Ethical Debate for Actuarial Risk Assessment. In Proceedings of the Conference on Fairness, Accountability, and Transparency, PMLR 81:62–76. [video] Masoud Mansoury, Bamshad Mobasher, Robin Burke, and Mykola Pechenizkiy. 2019. Bias Disparity in Collaborative Recommendation: Algorithmic Evaluation and Comparison. In Proceedings of the Workshop on Recommendation in Multi-stakeholder Environments at RecSys 2019. urn:arxiv:1908.00831v1 Alex Chohlas-Wood and E. S. Levine. 2019. A Recommendation Engine to Aid in Identifying Crime Patterns. INFORMS Journal on Applied Analytics, Vol 49, No. 2. doi:10.1287/inte.2019.0985. [preprint] Chen, I., Johansson, F. D. and Sontag, D. (2018) ‘Why Is My Classifier Discriminatory?’, in Bengio, S. et al. (eds) Advances in Neural Information Processing Systems 31. Curran Associates, Inc., pp. 3542–3553. No classes. Catherine D’Ignazio and Lauren Klein. 2020. “Introduction”, in Data Feminism. MIT Press. [online preprint] Ricardo Baeza-Yates. 2018. Bias on the web. Communications of the ACM (May 2018). doi:10.1145/3209581 Rishabh Mehrotra, James McInerney, Hugues Bouchard, Mounia Lalmas, and Fernando Diaz. 2018. Towards a Fair Marketplace: Counterfactual Evaluation of the trade-off between Relevance, Fairness & Satisfaction in Recommendation Systems. In Proceedings of the 27th ACM International Conference on Information and Knowledge Management (CIKM ’18). doi:10.1145/3269206.3272027 Javier Sánchez-Monedero, Lina Dencik, and Lilian Edwards. 2020. What does it mean to “solve” the problem of discrimination in hiring? social, technical and legal perspectives from the UK on automated hiring systems. In Proceedings of the 2020 Conference on Fairness, Accountability, and Transparency (FAT* ’20). doi:10.1145/3351095.3372849. [video] Flavio P. Calmon, Dennis Wei, Karthikeyan Natesan Ramamurthy, Kush R. Varshney. 2017. Optimized Data Pre-Processing for Discrimination Prevention. urn:arxiv:1704.03354. We will discuss this paper after the semester is over. Anna Lauren Hoffmann. 2019. Where fairness fails: data, algorithms, and the limits of antidiscrimination discourse. Information, Communication and Society. Routledge, 22(7), pp. 900–915. doi:10.1080/1369118X.2019.1573912.Background
Week 1 (Jan. 13–17)
Week 2 (Jan. 20–24)
Week 3 (Jan. 27–31)
Week 4 (Feb. 3–7)
Week 5 (Feb. 10–14)
Week 6 (Feb. 17–21)
Week 7 (Feb. 24–28)
Week 8 (Mar. 2–6)
Week 9 (Mar. 9–13)
Week 10 (Mar. 16–20)
Spring Break (Mar. 23–27)
Week 11 (Mar. 30–Apr. 3)
Week 12 (Apr. 6–10)
Week 13 (Apr. 13–17)
Week 14 (Apr. 20–24)
Week 15 (Apr. 27–May 1)
Week F (May 4–8)
Epilogue